COGNINTEL application experiment aims to deploy AI-based decision making and control platform tailored to the machine tools industry to optimize their operations by enabling an holistic and intelligent Quality, Energy and Maintenance (QEM) management approach.
Problem and Description
Currently the manufacturing industry uses Information Technology (IT), distributed sensors, embedded controls and big data analytics to manage and optimise separately KPIs such quality, energy efficiency, system availability and cost effectiveness. Therefore, each stage is an “island of efficiency”. Cognitive production will integrate these islands, enabling effective performance management throughout the plant. Cognitive production is enabled by AI techniques that extract value from information related to the on-line status of assets and deploy a decision-based self-learning platform able to react dynamically.
The COGNINTEL project aims to provide users and plant managers with the right tools to implement cognitive production by ensuring high production efficiency, high quality, minimising the use of resources while optimising maintenance strategies (avoiding safety related failures and minimizing process downtime).
SCORTA Srl as a leading Italian manufacturer of thread cutting tools, plays an important role in this context since the application of COGNINTEL tools represent a key product strategy to succeed in the post-Covid worldwide manufacturing market, by enabling the production of better products (thread cutting tool, taps) and also better services.
The technology provider ERREDUE Sas and the DIH CIIRC-CTU (Czech Institute of Informatics, Robotics, and Cybernetics of the Czech Technical University in Prague) support SCORTA in this experiment by providing proper AI and Machine-Learning solutions as well as replicating and validating the approach in complementary manufacturing scenarios (e.g. robotic applications) to boost future market uptake of COGNINTEL technologies.
AE Description
COGNINTEL relies on an integrated temporal domain (time series analysis) and spatial domain (unsupervised clustering) approach to sharply enhance diagnostic and prognostic capabilities while ensuring a quick deploy-ability, a high level of confidence and replicability/scalability.
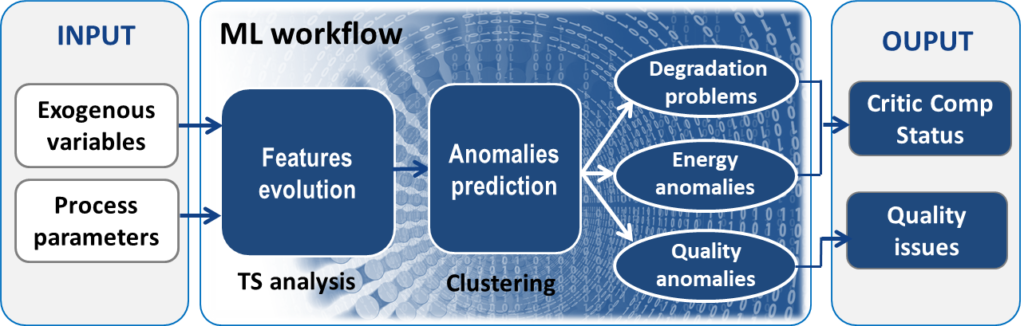
Up to now a TRL 4 has been reached: the activities implemented within this project regard the application experiment to prove the COGNINTEL concept in a relevant environment, then bringing the TRL up to TRL 6. In particular the key SW modules prototype (Learner and Predictor) of AI-based COGNINTEL platform will be improved to be fast deployed (short training time requested), flexible/scalable (modular approach), to ingest and cost-effectively process a consistent number of signal features (with a high level of confidence/accuracy).
AE Results and Impact
The experiment will deliver the following results to enable and prove a cost-effective deployment of CPES architecture/solutions:
- Physical layer (data collection, data fusion, and data communication/connection) implemented in demo facilities
- Cyber layer (data analytics and AI developments for highly confident diagnostics and prognostics, quick deploy-ability and a high level of replicability/scalability) implemented on a demo Server/Local (edge) workstation
- COGNINTEL platform validated against the defined KPIs for a CNC grinding/milling machine
COGNINTEL is an essential part of fostering rapid change and building resilience in manufacturing. Manufacturers with this digital platforms and advanced analytical capabilities will be able to react more quickly, effectively and successfully to Covid-19 disruptions. In particular COGNINTEL approach will:
- increase flexibility, reliability, sustainability and value for both base and disrupted operations by enabling our customers to monitor and analyse the status of the equipment remotely and to provide automatic alerts
- increase production efficiency and products quality while decreasing manufacturing cost
- leverage on AI and to capitalise on digitalization and foster smart and sustainable manufacturing
Partner & Expertise:
ERREDUE | Developing of AI/ML algorithms (Learner and Predictor modules). Support to implementation of solution in the end-user facilities. Final validation |
CIIRC-CTU | Lead and implement proper dissemination, communication and networking actions. Supporting industrial partners in replication test of the approach toward other industrial sectors |
SCORTA | Implementation of solution in a machining center for thread cutting tools production. Field data collection. Final validation |
For further information, please visit https://dih4cps.eu/experiment-14/